AIOps and Observability: What’s the Difference? | Riverbed
Interest in AIOps and observability tools sky-rocketed over the past couple of years as IT teams face the challenge of managing today’s IT infrastructures. The data explosion from modern architectures floods IT teams with massive volumes of data and alerts without context. Organizations are transforming and expanding service offerings into cloud-native, geographically distributed, container and micro-service-based architectures. Most are continuing to enable their employees to work remotely, requiring IT to support employees suffering from performance issues on home networks, on devices being run in sub-optimal conditions, and using SaaS and Shadow IT applications obtained outside of corporate IT.
The increase in complexity and volume of alerts is exacerbated by a shortage of highly skilled expert IT resources. Troubleshooting alerts requires expert IT staff to devote an excessive amount of time, taking them away from more strategic responsibilities.
Legacy monitoring can’t keep up with today’s IT environments
The situation is made worse by monitoring tools which alert on single metrics without broader context and correlation, lacking impact in scope and severity. IT teams have used legacy monitoring tools to collect data and generate alerts on violations of fixed or rate-of-change thresholds. Setting these alerts requires understanding the dependencies of the underlying infrastructure and what constitutes unacceptable performance. Monitoring is predicated on knowing in advance what signals you want to monitor (“known unknowns”).
In today’s modern, highly distributed architectures, it’s impossible for humans to understand these dependencies. Micro-service-based architectures spin up and down in cloud-native environments, so tracing topology after an incident doesn’t help. Too many factors outside of IT’s control affect the digital experience of an employee working from home for IT to resolve the issue. With proper credit to Donald Rumsfeld, there are too many “unknown unknowns.” These challenges have paved the way for observability and AIOps (Artificial Intelligence for IT Operations). Observability and AIOps are closely related, but there’s a difference between the two.
What is observability?
From systems control theory, observability is defined as the ability to measure the internal states of a system by examining its outputs. A system is considered “observable” if the current state can be estimated by using information only from outputs, namely sensor data.
In other words, building observability into a system eliminates the need to directly understand the dependencies of the underlying infrastructure, which can be treated as a “black box.” This is especially important in distributed systems like cloud-native environments, hybrid cloud networks, and even highly distributed remote work environments.
But observability is only as effective as the quantity and quality of the telemetry being provided. For IT to troubleshoot effectively, observability must include data across the full stack, including network, infrastructure, applications, digital experience, business key performance indicators (KPIs) and user sentiment. Unified Observability not only covers all these IT domains, but also captures metrics without sampling, so that full-fidelity data can be leveraged when resolving issues.
AIOps Market definitions
According to the Gartner Glossary, “AIOps combines big data and machine learning to automate IT operations processes, including event correlation, anomaly detection and causality determination.”
In the Forrester Now Tech: Artificial Intelligence for IT Operations, Q2 2022 (registration required), Forrester defines AIOps as “a practice that combines human and technological application of AI/ML, advanced analytics, and operational practices to business and operations data.”
AIOps platforms provide insights to IT staff by using AI and Machine Learning (ML) techniques to analyze telemetry and events from across the IT infrastructure and identify meaningful patterns that support proactive responses. In this way, AIOps platforms make the IT infrastructure observable to the IT teams involved in identifying and resolving issues.
The similarities between AIOps and observability
There’s a tight connection between AIOps and observability, but they are not the same. AIOps and observability have many common aspects and many vendors refer to their products as both AIOps platforms and observability platforms. So, market confusion is understandable.
- Similar business drivers. Business transformation is increasing the complexity of the underlying IT infrastructure of new applications and services that organizations are rolling out to better serve their customers. The integration of on-premises infrastructure and cloud services creates complex, ephemeral architectures that make it nearly impossible for humans to analyze and resolve issues.
- Shared customer requirements. IT teams need their operations from reactive to proactive. This goal is not new. IT has always striven to identify and resolve problems before end users are affected. But the increased dependence on digital performance has raised the stakes for greater availability and faster resolution times.
- Both evolve from traditional monitoring. Because of the limitations of traditional monitoring products addressed above, organizations are evolving to tools which incorporate AIOps and observability capabilities. Especially in response to the challenges of managing cloud-native environments. In response, vendors are evolving their Application Performance Monitoring (APM) products into both areas.
- Common use cases. Many products in the AIOps and observability segments address use cases for DevOps and site reliability engineering (SRE) teams. Again, traditional APM vendors have focused on use cases for these teams.
- Both are subject to over-hype. Both categories are listed in the “Peak of Inflated Expectations” according to Gartner Hype Cycle for Monitoring, Observability and Cloud Operations, 2022 (20 July 2022 ID G00770623, registration required)
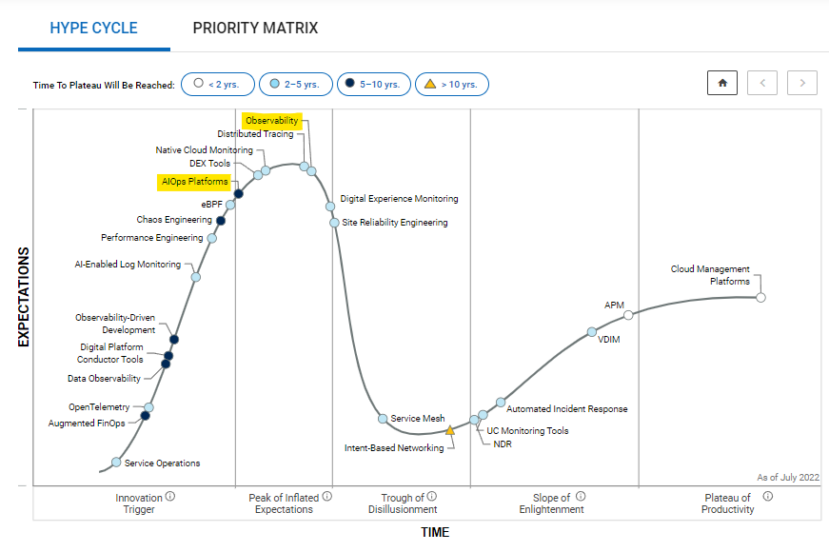
Both AIOps and Observability are shown in the “Peak of Inflated Expectations” in the Gartner Hype Cycle for Monitoring, Observability and Cloud Operations, July 2022, ID G00770623.
Riverbed’s focus: customer pain points
Confusion will continue to exist in the market about the difference between AIOps and observability. At Riverbed, we’re focused less on the specific names of market categories, and more on addressing the needs of our customers. Unlike other observability solutions that limit or sample data, Riverbed’s Alluvio Unified Observability portfolio captures full-fidelity user experience, application, and network performance data on every transaction across the digital ecosystem. It then applies AI and ML to contextually correlate data streams based on indicators of problems to provide actionable insights.
Our newest unified observability service, Alluvio IQ automates the investigative workflows of IT experts, empowering staff at all skill levels to solve problems, fast. With Alluvio IQ, IT can eliminate data silos, resource-intensive war rooms, and alert fatigue. They can enable cross-domain decision-making, apply expert knowledge more broadly, and continuously improve digital service quality. You can register for a complimentary evaluation today.
This post was first first published on Riverbed Blog’s website by Mike Marks. You can view it by clickinghere