The Importance of Automation in Data Classification
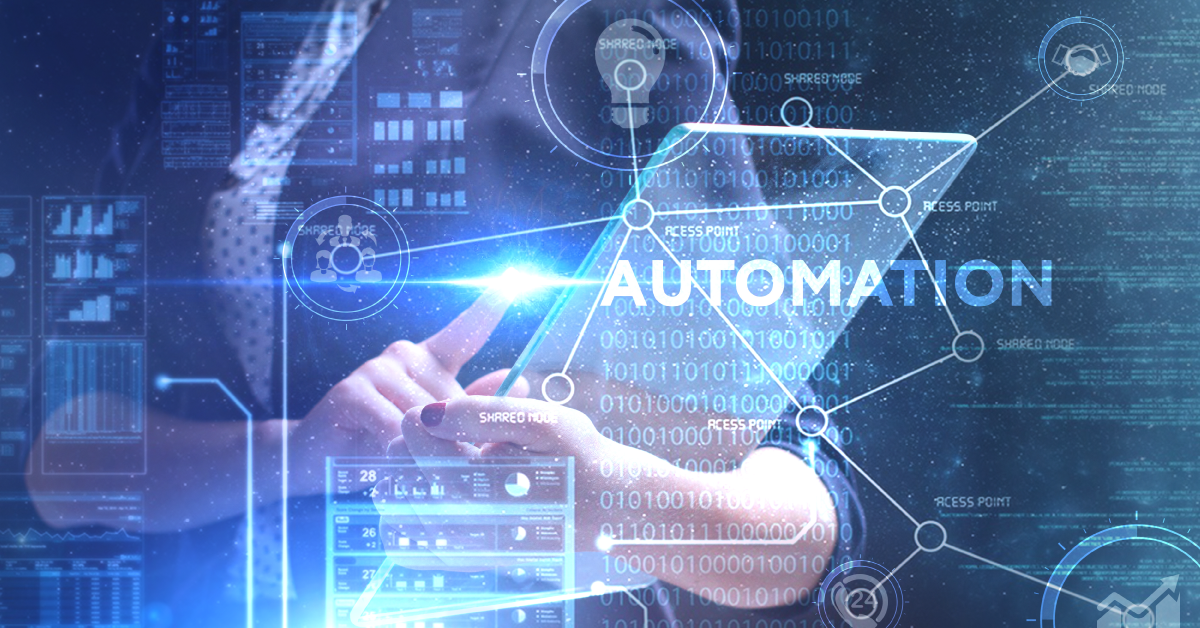
It’s no surprise to anyone that the amount of data that exists is rapidly growing. A report by IDC predicts that by 2025, the global datasphere will have grown to 175 zettabyes. To put in perspective how much data this truly is, one zettabyte is equal to one trillion gigabytes – that is an astronomical amount of data. Needless to say, humans are not equipped to manually keep up with ensuring this ever-expanding amount of data is appropriately classified and protected. This is where automated data classification comes into play.
How automated data classification works
Data discovery and classification for sensitive data allows an organization to identify where all its sensitive data resides and classify that data based on predetermined levels of sensitivity. Appropriate rich metadata is then applied to each document (including emails and Office documents) to inform your downstream security ecosystem, including the likes of data loss prevention (DLP) and cloud access security broker (CASB) solutions. While this may sound like a lot of required involvement from your users, automated data classification alleviates the burden by being programmed to automatically implement your organization’s classification policy as needed.
And while all of this is going on in the background, automated data security helps to keep security awareness front and center among staff. Every time data is created, shared, or otherwise handled, automated triggers and reminders based on the content or stakeholders involved appear, helping users to make informed and correct decisions when it comes to the classification level required.
Why automated data classification is important and why does an organization need it?
A study conducted by the Ponemon Institute found that only 23% of organizations used automation extensively (high automation organizations) while 77% of organizations used automation moderately, insignificantly, or not at all. They found that organizations who used automation extensively had six key benefits including the following relating to data classification and privacy:
- High automation organizations are more likely to say their organizations have the right number of security solutions and technologies. Automation can reduce complexity in the IT infrastructure. This can be accomplished by aligning in-house expertise to tools so that investments are leveraged properly.
- High automation organizations recognize the value of the privacy function in achieving cyber resilience and are also more likely to recognize the importance of aligning the privacy and cybersecurity roles in their organizations. These organizations recognize that the privacy role is becoming increasingly important, especially due to regulations such as GDPR and the California Consumer Privacy Act (CCPA).
Automated data classification solutions remind users to be cautious every time sensitive data is created, shared, or otherwise handled (even outright blocking that data’s dissemination to unauthorized parties, if necessary), extending a company’s security awareness program to even the most remote home office. And the best part? All this data discovery and classification for sensitive data can be automated, running in the background without end users being involved.
Blending automated and user-driven classification
There are many reasons an organization might choose to implement user-based classification, and there’s no doubt that this method works. But stepping into the world of automated classification can have huge benefits when it comes to ensuring your organization’s sensitive data is consistently classified and protected without having to rely on users’ manual input every time. Blending the use of automated techniques with user-driven data classification can deliver significant benefits including three key ones below:
- The delivery of a combined security approach that includes the user in the classification decision making process, improves awareness, and enhances overall security posture. By combining people, process, and technology, organizations can deliver on all key data protection and control requirements. Many organizations combine an automated and user-driven approach to provide an element of support to the user. An example of this could be the application of default labels based on user group or department. This approach reduces the number of clicks that the user must perform, but still involves users to ensure accuracy of the applied classification.
- The ability to integrate the rigor of technology-based automation alongside the contextual knowledge, use, and control requirements of data creators. Capturing user insight in the process of data classification is critical to ensuring decisions are made within the correct context. Data is constantly changing and can vary in sensitivity throughout its lifecycle and will likely need to be re-classified at some point. Automation can extend classification coverage across a variety of originating data sources, including those which originate outside of user control. This approach is useful when organizations have data generated by automated processes or systems that should be classified at the point of creation without user intervention. Then, if the data does need to re-classified manually at a later point in a specific context, it is easy to locate.
- The use of technology-based automation to assimilate knowledge about data and apply rule-based controls that fit the current and expected future needs of the organization without imposing additional operational overhead and expenses. Implementing automated data classification tools can significantly improve efficiency levels as the user involvement in manual document processing will be reduced. Automation can also reduce the possibility of human error, which is one of the biggest causes of accidental data breaches. Data classification solutions that utilize automation and machine learning for contextual analysis operate with an order of magnitude fewer errors.
The consequences of data exposure
Penalties for not complying with data privacy regulations are high, and can vary depending on an organization’s location, or the type of data they are dealing with. A recent survey by IBM showed that the average cost of a data breach among companies surveyed reached $4.24 million per incident in 2021, the highest in 17 years. In addition to the mitigation costs of a data breach (both financially and reputation-wise), there may also be penalties and fines due to regulations such as CCPA, GDPR, HIPAA, ITAR, and CUI.
The emergence of data protection regulations globally is rapidly increasing, with many regulations following suit from the implementation of GDPR in 2018. A recent article in The National Law Review details that 23 states in the USA introduced some form of all-encompassing data privacy legislation to address the absence of federal privacy laws in 2021, with two becoming law in Virginia and Colorado. Privacy regulations are also emerging in India as the revised draft of the Personal Data Protection Bill (PDP) was submitted to Parliament on December 16th, 2021. These are just a few examples of how data privacy regulations are emerging worldwide. Ongoing, organizations will need to pay extra attention to these emerging privacy laws and make sure they are adequately equipped to secure data they are creating and storing to avoid the risk of crippling fines and reputational damage.
By involving automation in the data classification process, organizations are working smarter to provide consistent protection, meaning they can stay one step ahead of these emerging regulations. As data volumes and privacy regulations continue to grow, maintaining the confidentiality, integrity, and availability of data has become a priority for all organizations. Automation plays a central role in data governance and helps to maintain the required balance between technology and people-focused operations.
With the volume of data created on a daily basis continuing to rise, organizations should be looking to implement data identification and classification tools that can work in a scalable and accurate manner, enabling safe storage, sharing, and analytics. By combining people, process, and technology, organizations can deliver on all key data protection and control requirements; not only with regard to ensuring understanding and appropriate management of data, but delivering the breadth of security coverage required on a local and remote basis.
This post was first first published on Titus website by Elizabeth Swabey-Keith. You can view it by clicking here